The concept of computers surpassing human capabilities should come as no surprise. The magnitude of this discrepancy, however, is not always appreciated. The speed of modern desktop processors is commonly measured in gigahertz – that is, the number of clock cycles completed in the order of billions. To put this into perspective, someone who could list three numbers a second would take over 10 years to reach the first billion. Increasingly accessible hardware is indisputably providing the capacity to tackle more complex problems in an ever-diminishing period of time and cost.
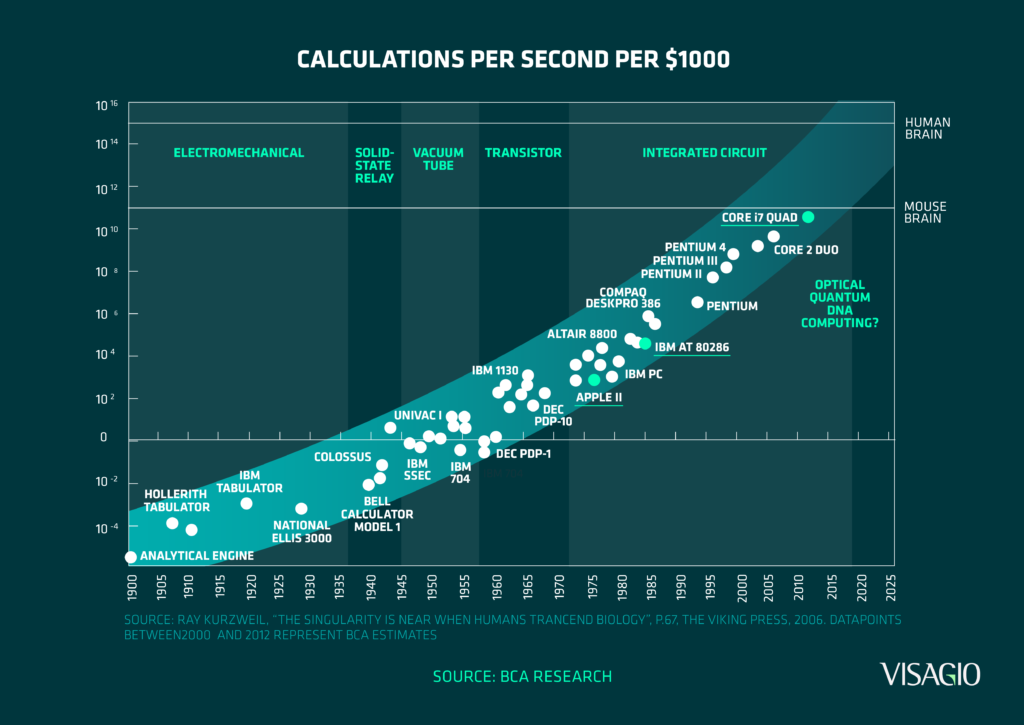
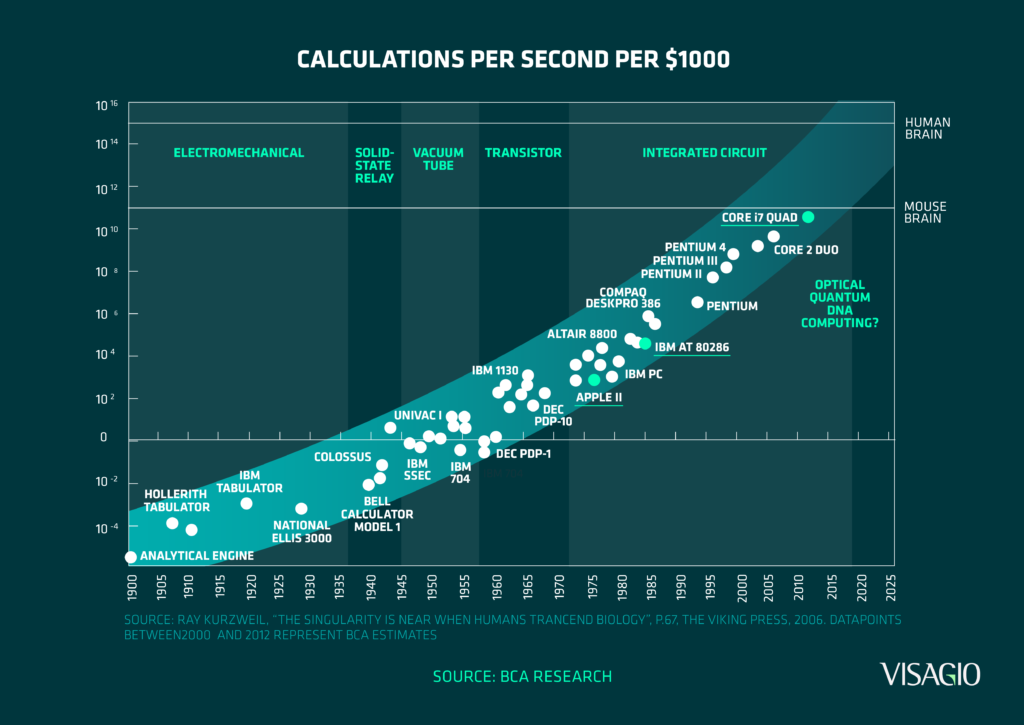
The exponential advancements in processors over time
A seemingly unlikely example of this can be found within biological research – in particular, the area of genome sequencing. For human genomes, the process consists of transcribing the roughly 6 billion long code of letters that defines a person’s DNA. The difference in costs for completing this task over the past years clearly highlights the continual advancements in technology and its capabilities.
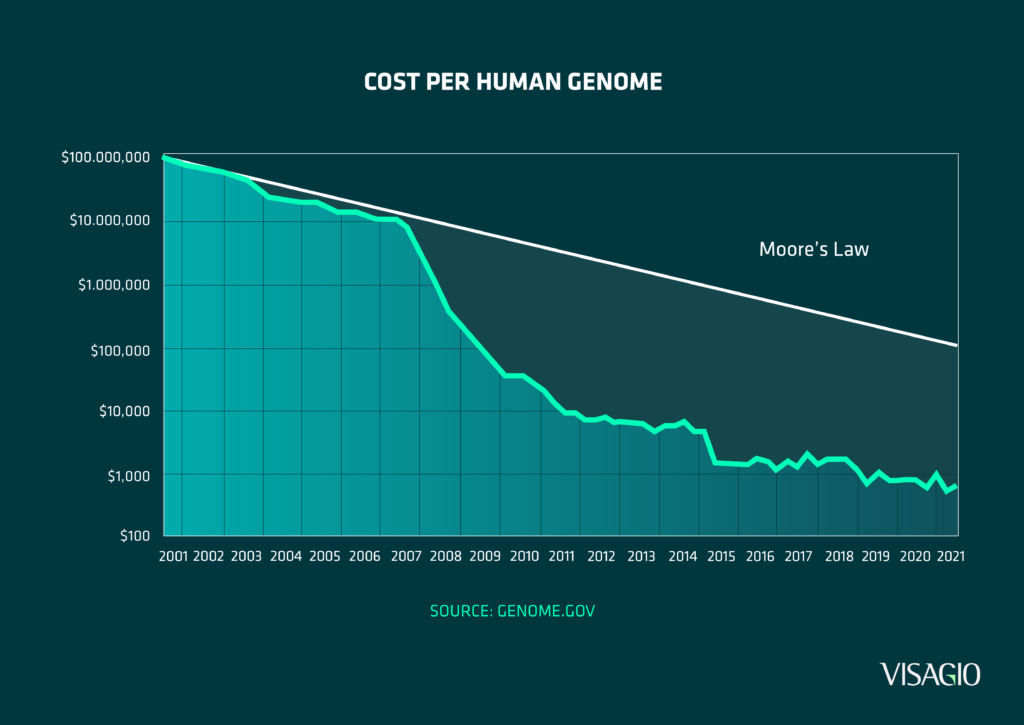
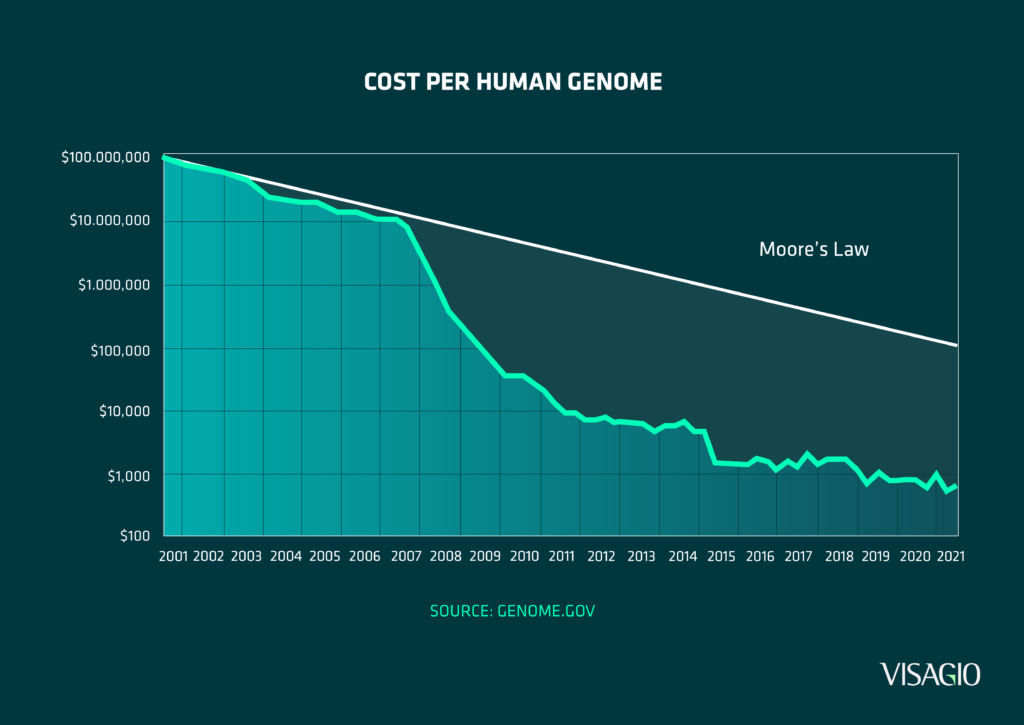
An application of the increasing computational capacity in the genetic field
With this ever-growing computational power, a new challenge arises on how to expand our analytical landscape to fully utilise this potential. While the opportunities are virtually endless, many continue to be realised; in a time where industries continue to lean further into digital technologies and invest in vast centres of data, it is necessary to seize the opportunities presented in order to effectively operate and innovate at a leading pace.
Digital Applications
Operations research (OR) is one of many disciplines that capitalises on the growing potential of technology, aiming to apply advanced analytics and modelling to augment decision-making. As digital advisors, applications of OR combine mathematical concepts with the processing strength of modern machines to solve problems that exceed manual capacity. These fields span a variety of studies, including optimisation, simulation, and forecasting. As industries move towards the next levels of digital maturity, the importance of this research becomes increasingly apparent.
Over the past 300 years, three industrial revolutions have been noted – the first was the incorporation of machines in production with steam power, the second saw the expansion of connectivity and technological networks to mechanise production lines, and the third revolution marked the adoption of supercomputers.
The fourth industrial revolution is believed to be occurring right now. The ever-approaching “Industry 4.0” is often characterised by several things like autonomous systems, industrial Internet of Things, and big data but underpinning them are the key pillars of comprehensive data collection, data governance and decentralisation of decisions [1]. Despite the generation of data being a focus for many over the past years, only a fraction of it was being used to drive the decisions and processes they result from. Effective utilisation of data is a defining factor of the ability for many companies to capitalise on their assets.
At the core of this technological revolution sits advanced analytics like OR. The ability to provide a deeper understanding of systems, predict their future behaviour and estimate the impacts of changes are significant levers within the decision-making process focused on by these disciplines. The bridge connecting the information captured on one side to the informed actions being executed on the other is critical to building a successful Industry 4.0 ecosystem.
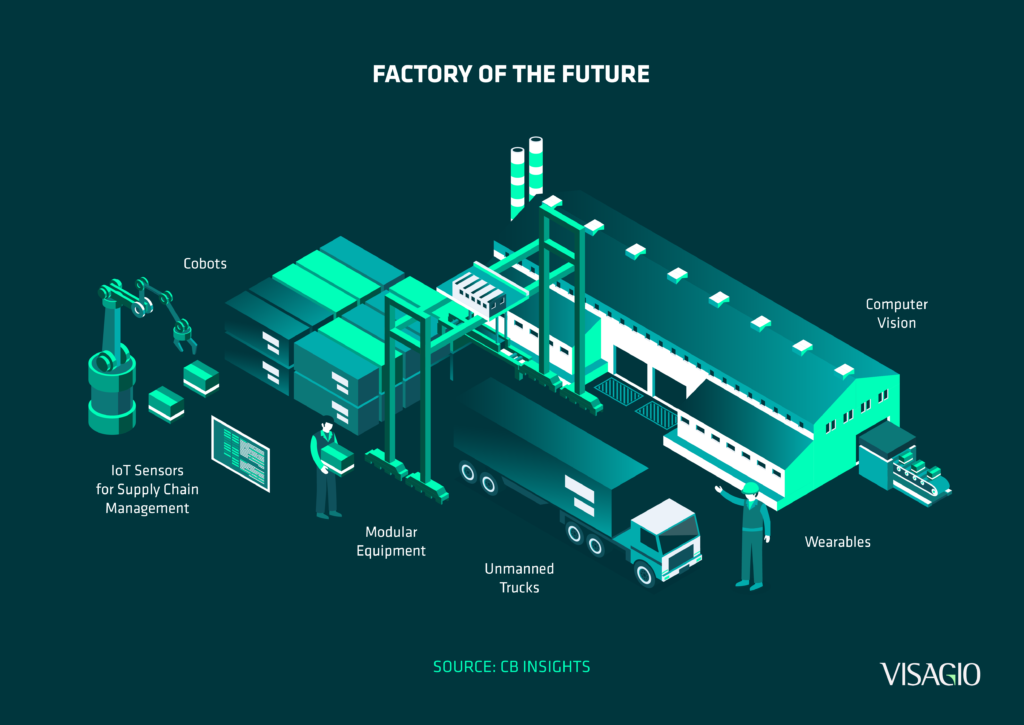
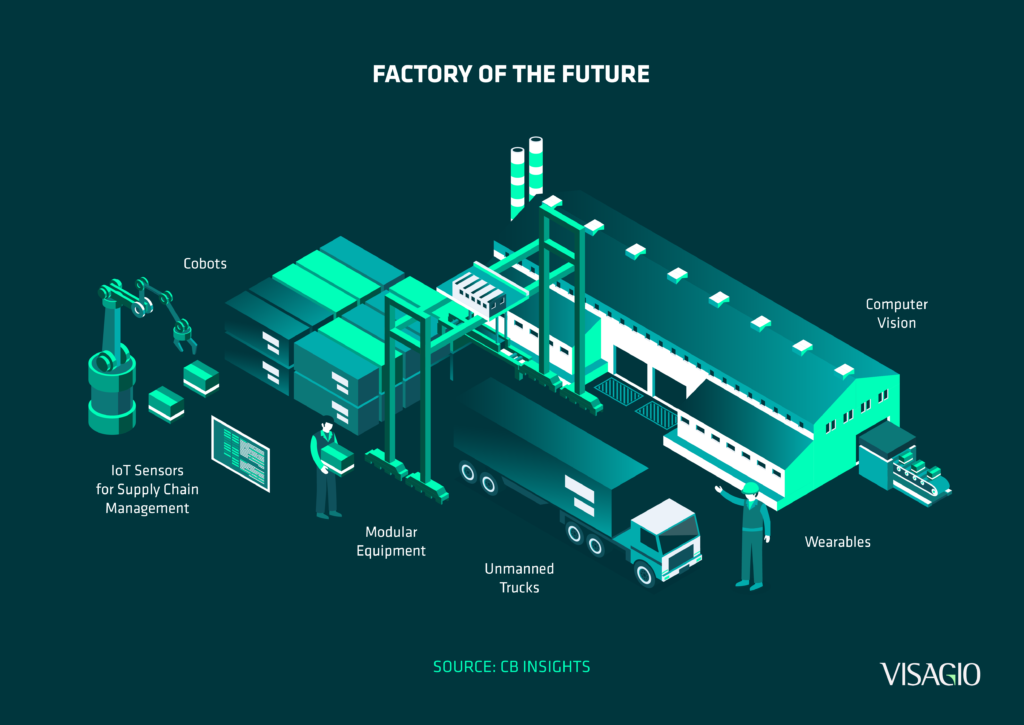
Key technologies of Industry 4.0 forming a smart factory
However, a fundamental component to these analyses is the data being supplied to them. This is reflected in the principles of ensuring the capture of high-quality information and its accessibility within the Internet of Things. A result’s accuracy is contingent on the inputs to the analysis, while its complexity is a product of the input’s breadth and depth. As an extension of that, sectors such as mining and economics that produce large, detailed datasets stand to benefit the most by maximising the use of OR. A more in-depth discussion on this topic and high-level breakdown of the structure of OR models can be found here.
Digitalising a Supply Chain
Supply chain management and planning provide a key use case for these analytics. The supply chain’s structure naturally translates into a mathematical model of nodes and edges, allowing for applications of techniques to provide further insight into the network. One such example is through simulation–modelling system behaviours and their interdependencies. Once the properties of the supply chain are fully mapped and represented, circumstances can be flexed to test a variety of scenarios and provide accurate results of performance – answering questions such as the impacts on overall throughput or shifts in the bottleneck through the introduction of new equipment or reduction in failure rates.
Optimisation is another area of research that is often grouped with simulation but has key differences. While the former aims to provide the most realistic result given certain inputs, the latter looks to find the best outcome given a particular system and its constraints. For a supply chain, both are powerful analyses due to the importance of understanding reactions to changes in processes or design and how best to utilise the current network.
In many businesses, however, supply chain planning continues to be a manual process. Traditionally, the expertise and intuition of an experienced team are relied upon over several sessions to produce a schedule for the upcoming time horizon. Despite this effort, the scale and complexity of the task often require heuristic rules, generalisations, and simplifications for practicality – at the cost of system efficiency.
In mine networks consisting of shovels, crushers, and interwoven conveyors, the number of potential combinations exponentially grows to the order of tens of thousands. While condensing these to a predefined set of routes can be an effective alternative through careful selection, the flexibility and capacity of the supply chain will be unavoidably hindered – more so in the complex and densely interconnected networks that require simplifications the most.
Adding a further layer of complexity to the individual route choices, it is essential to consider how these decisions affect the network further down the stream. Quality standards, equipment capacity and production rates are all examples of factors that can be influenced through material routing and blending. A holistic view of both the supply chain and the planning timespan is required for each choice to accurately develop a plan to its full value. However, due to the scale of these networks, their myriad of components and the cascading impact of each choice over an extended timeframe, calculating them manually is simply not possible.
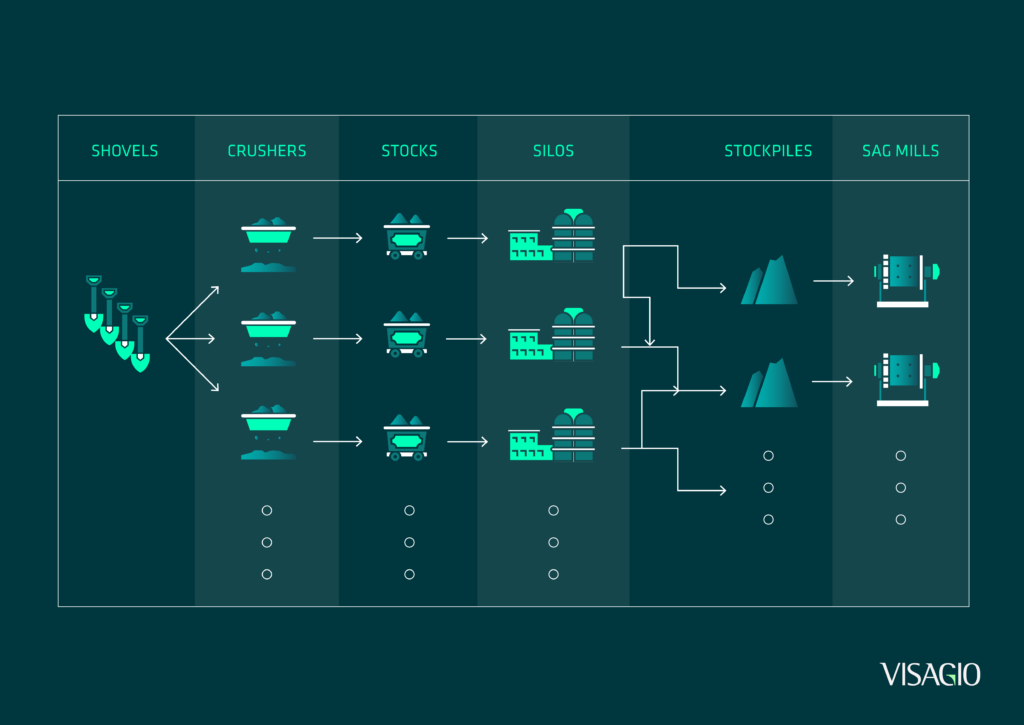
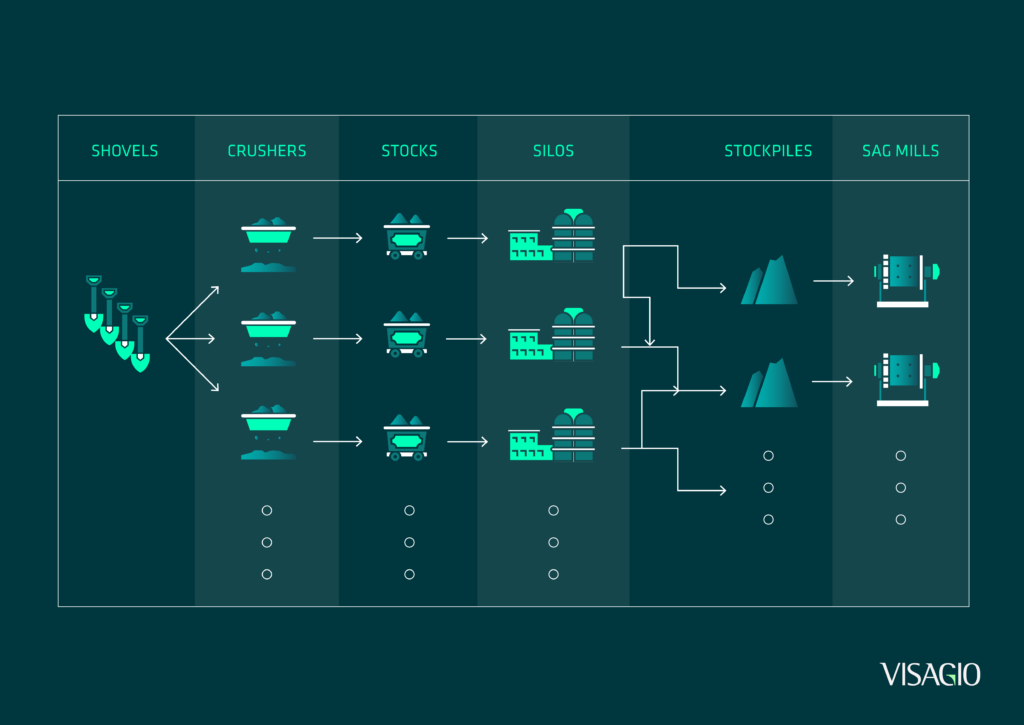
The branching and interlocking paths of a mine supply chain
In contrast, readily available optimisation software are capable of processing several million variables at a time. The initial investment of time in the implementation of an optimiser is offset by the ensuing calculations themselves being highly optimised as a result of the years of research and technological advancements preceding them. To understand the scale of this evolution, there are optimisation problems now solvable in one second that would have taken 55 years to solve in 1991 – solvers that would still be running to this day.
The benefits of an optimiser’s speed come in two key areas. Firstly, the team involved in planning would be able to focus on more value-adding tasks, their subject matter expertise embedded into the model’s construction and definition. Secondly, the calculation’s efficiency unlocks a level of repeatability previously infeasible. Optimisations can be computed across various configurations to map potential options and compare the optimal results of different scenarios. Additionally, they can be rerun to adapt to unforeseen changes – such as critical equipment failure or expansions in the supply chain – which would previously require a reassembly of planners. Multiple tests can be carried over a range of possibilities to further contribute to risk reduction along with the generation of multiple plan options to enrich the strategic environment.
Most apparently, a major advantage of using an optimiser is its output – computing the theoretically optimum plan for a defined system. The margin of improvement depends largely on the complexity of the supply chain and the extensiveness of previous planning. However, the analyses also add a further layer of confidence to the designs by avoiding mass simplifications and intuition in favour of mathematical programming and historical trends. In a recent paper by several consultants from Visagio [2] on a copper mine network, the site saw a potential increase of 4.47% in throughput by applying the optimisation plans over previous performance under manual scheduling. This improvement, as defined in the model, involves the same network as before and purely stems from the utilisation of existing equipment to a greater extent. This highlights the effectiveness of more complex computations. The simplifications required by the limitations of manual planning resources prevented fully realising the potential.
Conclusion
Applications of simulation and optimisation as two of many fields in Operations Research, offer opportunities to augment processes and decision-making. In supply chains, the computational capabilities of modern computers overcome the generalisations and simplifications of the past that were required to make the problem feasible. Furthermore, incorporating advanced analytics into routines show improvement in performance with better utilisation of existing assets. The planning pipeline is simultaneously de-risked through repeatability in response to change or by providing alternative options, an advantage previously limited by the reliance on the team’s manual work and its time-consuming nature.
Ultimately, this is made possible through the union of mathematical concepts and the evolution of processing technology – a potential that continues to increase as the industry 4.0 landscape advances over the coming years. As the world progresses further into the digital age, it is imperative to vigilantly identify ways to improve and augment the status quo – whether that be digitalising manual processes or informing designs through advanced analytics. While applications of technology have eclipsed human processing capacities, they may soon do the same to what we currently believe to be the extent of their potential.
References
[1] Klaus Schwab (2016). The Fourth Industrial Revolution. World Economic Forum
[2] Luan Mai, Zenn Saw, Carlos Poveda. (2021). Optimisation of pit to SAG mill network using mixed integer programming. Paper presented at the APCOM 2021, South Africa
About the author
Zenn Saw is a Visagio consultant with experience in Optimisation, Cloud Computing and Data Analysis with a contextual focus in the mining industry. He comes from a background in Computer Science and Mathematics & Statistics, having studied a double major at the University of Western Australia. He has recently been involved in a project to drive digital acceleration within a tier 1 mining company by expanding the analytical toolkits and improving current processes.